THIS ARTICLE AT A GLANCE
CONTACT ETS
If you have any questions or would like to discuss further what you should be doing, ETS is here and willing to help.
Call 0117 205 0542
Email enquiries@energy-ts.com
Submit a contact form
CHECK OUR SERVICES
Measuring Energy Consumption
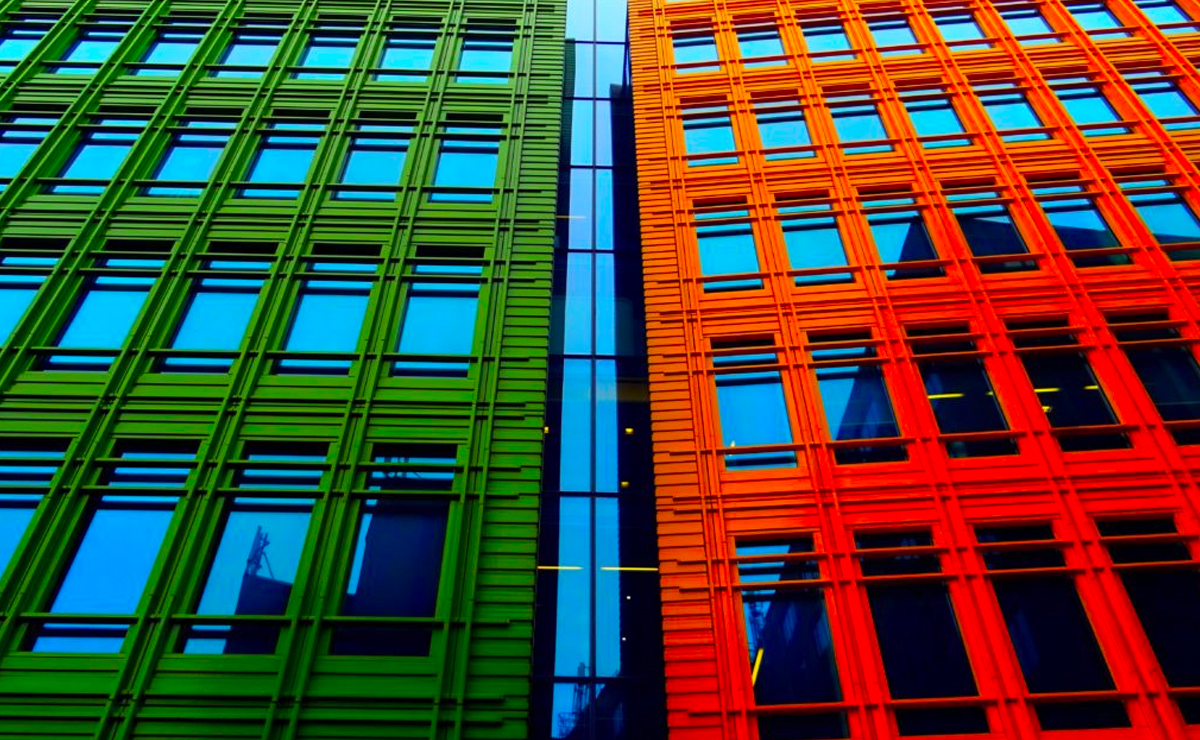
For many people, the beginning is the time of year for resolutions and target-setting. So what better time to consider how we measure how well we are doing?
As energy managers, we face this problem all year round when it comes to measuring energy consumption. Our first challenge is to understand how much energy we are using, and our second challenge is to establish whether that energy consumption is reasonable or not. We do this through the regular practice of Monitoring and Targeting (M&T).
M&T relies on the comparison of actual energy consumption to a reference consumption (or baseline) for the same time period. When these values are compared, any differences between the actual consumption and reference consumption are highlighted. These differences can help identify equipment failure, poor control, or other causes of energy waste. But the quality of outputs from an M&T programme are heavily reliant on having an accurate baseline to compare against. So where do these baselines come from?
Two Models for Monitoring and Measuring Energy Consumption
ASHRAE (American Society of Heating, Refrigeration and Air-Conditioning) distinguishes two basic ways to create a baseline when measuring energy consumption: “Forward Models” are based on known users of energy, such as a schedule of lighting loads, or a building’s heat-loss characteristics; “Inverse Models” meanwhile, have no interest in what is using the energy, but instead, rely on patterns of historic consumption to predict future energy use.
“Inverse” or historic-based models are certainly the easiest to create. As a first iteration, you can simply compare one week’s data to the next, to see if it is higher or lower. You might compare day-to-day or year-to-year, but whatever the time period, your baseline is the historic data. The increasing prevalence of energy management software is making historic-based comparison ever more popular, since it’s a relatively simple function to provide. When energy software packages offer targeting and alert functionality, this is nearly always based on whether the data is better or worse than a comparable period in the past.
More sophisticated inverse models use regression analysis to identify how energy use is influenced by factors such as air temperature, occupancy, or production volumes. This can be very useful as a way to use historic performance as a baseline, even when conditions for both periods are not completely identical. For example, an energy manager might use Heating Degree Days to create an energy baseline that is lower in warm weather and higher in cold weather. Machine-learning models do this very well, and can “predict” expected energy use to a high level of accuracy.
But all historic-based models have one major flaw. All they can tell you is whether you are better or worse than you used to be. If your energy consumption has always been higher than it should be, you are likely to perpetuate that poor performance. As Shirley Bassey would say, “It’s all just a little bit of history repeating”.
Implementing a Forward Model
The best way to avoid this problem is to consider the “forward model” approach. Forward models don’t need any historical data to run. Instead, they use information about the building fabric, lighting, and HVAC to compute the energy flows in and out of the building, and predict the energy requirements. The dynamic building simulation software required to create a detailed forward model is highly complex and can demand thousands of inputs. But for many buildings, even a rough estimate of full-load energy based on a simple asset inventory can give some really valuable insights.
As part of a recent study into a group of near-identical supermarkets, ETS created three different baselines to investigate which one was most helpful for energy management. The first was based on a historic baseline, using regression techniques to adapt to changes in outdoor temperature and occupancy. The second was a simple average of the consumption across all of the sites. The final baseline was a detailed dynamic building simulation.
The historic baseline performed best at predicting actual consumption. It remains the simplest and best approach for highlighting occasional deviations from normal operating patterns, particularly where the variables driving energy use are well understood.
However, the building simulation baseline and the peer-group baseline showed up a number of energy management issues that were not apparent from the historic view. These findings included a long-term problem with control of lighting overnight, excessive (and unexplained) exterior lighting loads, and an unusual sensitivity to outdoor air temperature for refrigeration at one of the sites.
On their own, none of the baseline approaches were able to highlight all of the energy-saving opportunities that were present. So, if your new year resolution is to achieve more energy savings, consider whether a new baseline approach might just show you a new way to measure energy consumption and highlight areas for improvement.
Final thoughts
If you are looking for an energy management system that is tailored to your business needs, ETS can provide you with 25 years of experience in dramatically improving energy efficiency and reducing environmental impacts. Whether your businesses have individual assets or large international portfolios, ETS can assist you in saving substantial amounts of money while significantly reducing your carbon performance.
To discuss your requirements, get in touch. You can contact us by calling 0117 205 0542 or drop us an email at enquiries@energy-ts.com.
For many people, the beginning is the time of year for resolutions and target-setting. So what better time to consider how we measure how well we are doing?
As energy managers, we face this problem all year round when it comes to measuring energy consumption. Our first challenge is to understand how much energy we are using, and our second challenge is to establish whether that energy consumption is reasonable or not. We do this through the regular practice of Monitoring and Targeting (M&T).
M&T relies on the comparison of actual energy consumption to a reference consumption (or baseline) for the same time period. When these values are compared, any differences between the actual consumption and reference consumption are highlighted. These differences can help identify equipment failure, poor control, or other causes of energy waste. But the quality of outputs from an M&T programme are heavily reliant on having an accurate baseline to compare against. So where do these baselines come from?
Two Models for Monitoring and Measuring Energy Consumption
ASHRAE (American Society of Heating, Refrigeration and Air-Conditioning) distinguishes two basic ways to create a baseline when measuring energy consumption: “Forward Models” are based on known users of energy, such as a schedule of lighting loads, or a building’s heat-loss characteristics; “Inverse Models” meanwhile, have no interest in what is using the energy, but instead, rely on patterns of historic consumption to predict future energy use.
“Inverse” or historic-based models are certainly the easiest to create. As a first iteration, you can simply compare one week’s data to the next, to see if it is higher or lower. You might compare day-to-day or year-to-year, but whatever the time period, your baseline is the historic data. The increasing prevalence of energy management software is making historic-based comparison ever more popular, since it’s a relatively simple function to provide. When energy software packages offer targeting and alert functionality, this is nearly always based on whether the data is better or worse than a comparable period in the past.
More sophisticated inverse models use regression analysis to identify how energy use is influenced by factors such as air temperature, occupancy, or production volumes. This can be very useful as a way to use historic performance as a baseline, even when conditions for both periods are not completely identical. For example, an energy manager might use Heating Degree Days to create an energy baseline that is lower in warm weather and higher in cold weather. Machine-learning models do this very well, and can “predict” expected energy use to a high level of accuracy.
But all historic-based models have one major flaw. All they can tell you is whether you are better or worse than you used to be. If your energy consumption has always been higher than it should be, you are likely to perpetuate that poor performance. As Shirley Bassey would say, “It’s all just a little bit of history repeating”.
Implementing a Forward Model
The best way to avoid this problem is to consider the “forward model” approach. Forward models don’t need any historical data to run. Instead, they use information about the building fabric, lighting, and HVAC to compute the energy flows in and out of the building, and predict the energy requirements. The dynamic building simulation software required to create a detailed forward model is highly complex and can demand thousands of inputs. But for many buildings, even a rough estimate of full-load energy based on a simple asset inventory can give some really valuable insights.
As part of a recent study into a group of near-identical supermarkets, ETS created three different baselines to investigate which one was most helpful for energy management. The first was based on a historic baseline, using regression techniques to adapt to changes in outdoor temperature and occupancy. The second was a simple average of the consumption across all of the sites. The final baseline was a detailed dynamic building simulation.
The historic baseline performed best at predicting actual consumption. It remains the simplest and best approach for highlighting occasional deviations from normal operating patterns, particularly where the variables driving energy use are well understood.
However, the building simulation baseline and the peer-group baseline showed up a number of energy management issues that were not apparent from the historic view. These findings included a long-term problem with control of lighting overnight, excessive (and unexplained) exterior lighting loads, and an unusual sensitivity to outdoor air temperature for refrigeration at one of the sites.
On their own, none of the baseline approaches were able to highlight all of the energy-saving opportunities that were present. So, if your new year resolution is to achieve more energy savings, consider whether a new baseline approach might just show you a new way to measure energy consumption and highlight areas for improvement.
Related Article
8 Ways Businesses Can Reduce Energy Use in the Workplace This Winter
Discover how to comply with ESOS Phase 4 and unlock energy-saving opportunities for your business. This guide explains the requirements, highlights key deadlines, and provides actionable strategies. Learn how energy audits, tailored action plans, and expert support can reduce costs, improve efficiency, and align your organisation with sustainability goals.
ESOS Action Planning: Complying with Phase 4 and Implementing Energy Saving Strategies
Discover how to comply with ESOS Phase 4 and unlock energy-saving opportunities for your business. This guide explains the requirements, highlights key deadlines, and provides actionable strategies. Learn how energy audits, tailored action plans, and expert support can reduce costs, improve efficiency, and align your organisation with sustainability goals.
Important Update: What You Need to Know about ESOS Phase 3
Time is ticking for the ESOS Phase 3 deadline. The Environment Agency announced that the reporting system is available now. For organisations qualifying for ESOS Phase 3, the deadline for submitting a compliance notification is 5 June 2024, and organisations should still look to meet this compliance notification deadline where possible.